That might also interest you
CKDNapp: A toolbox for monitoring and tailoring treatment of chronic kidney disease patients - a personalized systems medicine approach
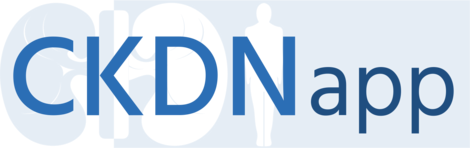
Aims of the project
Chronic Kidney Disease (CKD) is a disease of multiple causes. It is characterized by a variable course of diseases and a high burden of cardiovascular (CV) and metabolic comorbidities, complicating optimal treatment.
To provide optimal, personalized medical care for each patient, physicians need to obtain a detailed, overall picture of that patient’s state and therapy decisions derived from that.
For this purpose, he/she integrates different levels of data, e.g., clinical/demographic parameters, biomarkers, and drug information, with medical knowledge.
Because CKD is a complex disease, this data integration process is extremely challenging.
The collaborative aims are:
1. to computationally model this complex CKD system
2. to enrich these models with novel omics data
3. to discover novel biomarkers and
4. to build a clinical decision support (CDS) software based on these models assisting physicans in personalized everyday CKD patient care
The CDS software, called CDKNapp (CKD Nephrologists'app) will predict adverse medical events and disease progression, refine diagnosis of CKD staging, return transparent reasoning for all predictions and recommendations, offer in silicio modification of patient parameters by the physican, and will deliver comprehensive literature support. It will be made available as an easy-to-use software for smartphones, tablets and desktop computers.
Contribution of the Department of Medical Bioinformatics
The Department of Medical Bioinformatics is involved in the subproject 3 and 4.
Subproject 3: Algorithmic foundation of CKDNapp
PI: Prof. Dr. Michael Altenbuchinger
The Chronic Disease Nephrologist's App (CKDNapp) is designed as a clinical decision support system to assist the practising nephrologist in the management of patients with chronic kidney didease. The software uses the complex relationship between a variety of patient parameters, such as the patients's age, laboratory parameters and gender. Learning such relationships is a typical problem in machine learning.
In this subproject, customised algorithms will be developed for this purpose, which will then serve as a basis for the development of CKDNapp. In addition, methodology will be developed to extract metabolite concentrations from metabolite profiles generated from blood samples of the patient with kidney disease using NMR spectroscopy. These concentrations are a rich source to investigate possible effects of the kidney disease on the patient's metabolism. All developed algorithms will finally be made available to the public as user-friendly software.
Subproject 4: CKDNapp application and web service development
PI: Dr. Jürgen Dönitz (previous Dr. Johannes Raffler)
The final goal of the CKDNapp project is to support local nephrologist to choose the best treatment for the single patients. The models developed by the other project partners will be completed with other public data, literature and guidelines in an interactive application.
The project is fundet by the BMBF.
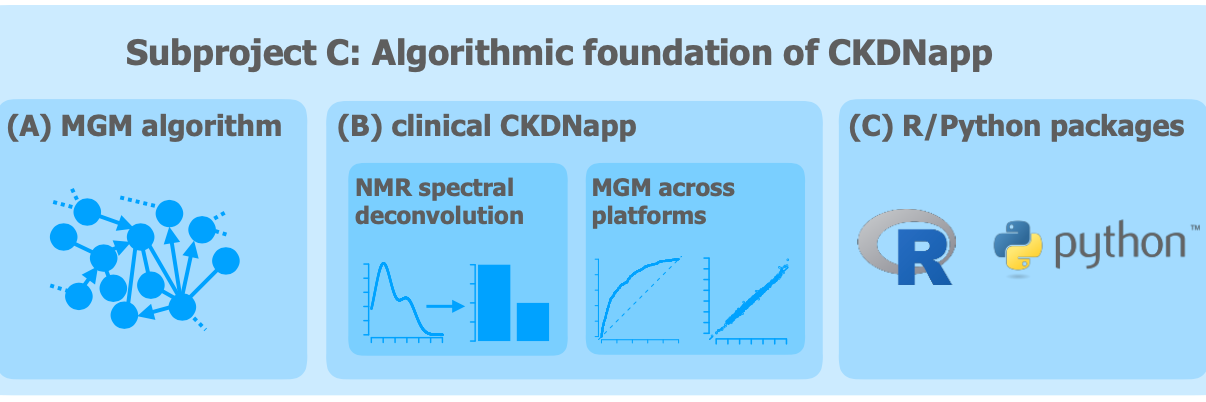
Publications
Zacharias, H. U., Altenbuchinger, M., Schultheiss, U. T., Raffler, J., Kotsis, F., Ghasemi, S., ... & Oefner, P. J. (2021).
A Predictive Model for Progression of CKD to Kidney Failure Based on Routine Laboratory Tests.
American Journal of Kidney Diseases.
Altenbuchinger, M., Zacharias*, H. U., Solbrig, S., Schäfer, A., Büyüközkan, M., Schultheiß, U. T., Kotsis, F., Köttgen, A., Spang, R., Oefner, P. J., Krumsiek, J., Gronwald, W. (2019). A multi-source data integration approach reveals novel associations between metabolites and renal outcomes in the German Chronic Kidney Disease study.
Scientific Reports, 9(1), 1-13.
Zacharias, H. U., Altenbuchinger, M., Schultheiss, U. T., Samol, C., Kotsis, F., Poguntke, I., Sekula, P., Krumsiek, J., Köttgen, A., Spang, R., Oefner, P. J., & Gronwald, W. (2019).
A Novel Metabolic Signature To Predict the Requirement of Dialysis or Renal Transplantation in Patients with Chronic Kidney Disease.
Journal of Proteome Research, 18 (4), 1796-1805.
Coordination and Project partners
Coordinator:
Prof. Dr. Helena U. Zacharias
Universitätsklinikum Schleswig-Holstein
Klinik für Innere Medizin
AG Klinische Metabolomik
Kiel
Project Partners:
Dr. Ulla Schultheiß
Albert-Ludwigs-UniversityFreiburg
Faculty of Medicine
University Medical Center
Institute for Genetic Epidemology
Prof. Dr Michael Altenbuchinger
University Medical Center
Department of Medical Bioinformatics
Dr. Johannes Raffler (until 2021)
Helmholtz Zentrum München
Deutsches Forschungszentrum für Gesundheit und Umwelt
Institute of Computational Biology (ICB)
Dr. Jürgen Dönitz (since 2021)
University Medical Center
Department of Medical Bioinformatics