Medical Data Science
Background
Data sciences and machine learning in medical research
Research in medicine changed substantially in recent years. Modern high-throughput technologies, such as RNA sequencing provide data of unprecedented amount and quality. Multiple molecular layers of information, such as the transcriptome and methylome, can be assessed within individual cells, and ven with spatial resolution.
These new data provide a valuable resource to gain insights into diseases and their patho-mechanisms. Computationally, methods have to deal with an overwhelming complexity. High-dimensional statistics and machine learning can help to resolve this complexity. However, yet, appropriate methods are rare, and need to be futher developed or adapted to deal with new, upcoming biomedical data.
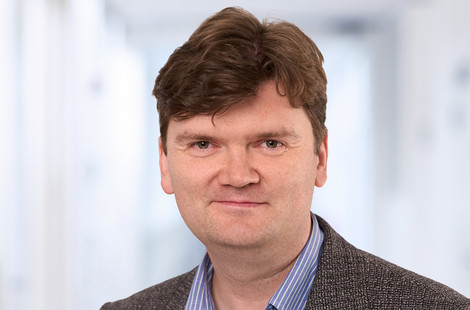
Research Foci
We focus on developing computational approaches to deal with new, upcoming high-throughput data in the context of biomedical research. This comprises the development of machine learning methods for clinical decision support, and for the inference of molecular networks and mechanisms. Further, we are highly interested in applying state-of-the-art methods to solve questions that arise within research collaborations. Here, our focus is cancer research and kidney metabolomics.
Current projects
FDLP: Federated Learning in Lymphoma Pathology (2022 - 2024)
This poject supports the development of federated machine learning methods to develop models that enable the prediction of prognostic subtypes. For more information see the project site or the BMBF project site.
DTD: Digital Tissue Deconvolution
In this project, we develop computational methods to estimate cellular compositions from bulk gene-expression data. For more details, see the project site. More...
CKDNapp: The Chronic Kidney Disease Nephrologist’s App
In this project we develop and apply computational methods to improve the treatment of patients with chronic kidney disease. For more details, see the project site. More...
Selected publications
Zacharias, H. U., Altenbuchinger, M., Schultheiss, U. T., Raffler, J., Kotsis, F., Ghasemi, S., ... & Oefner, P. J.
A Predictive Model for Progression of CKD to Kidney Failure Based on Routine Laboratory Tests.
American Journal of Kidney Diseases. 2021
Jachimowicz, R. D., Klapper, W., Glehr, G., Müller, H., Haverkamp, H., Thorns, C., ..., Altenbuchinger, M., Rosenwald, A.
Gene expression-based outcome prediction in advanced stage classical Hodgkin lymphoma treated with BEACOPP.
Leukemia, 1-5. 2021
Staiger, A. M., Altenbuchinger, M., Ziepert, M., Kohler, C., Horn, H., Huttner, M., ... & Spang, R.
A novel lymphoma-associated macrophage interaction signature (LAMIS) provides robust risk prognostication in diffuse large B-cell lymphoma clinical trial cohorts of the DSHNHL.
Leukemia, 2020, 34(2), 543-552.
Görtler, F., Schoen, M., Simeth, J., Solbrig, S., Wettig, T., Oefner, P. J., ... & Altenbuchinger, M.
Loss-function learning for digital tissue deconvolution.
Journal of Computational Biology, 2020, 27(3), 342-355.
Altenbuchinger, M., Rehberg, T., Zacharias, H. U., Stämmler, F., Dettmer, K., Weber, D., ... & Spang, R.
Reference point insensitive molecular data analysis.
Bioinformatics, 2017, 33(2), 219-226.
Altenbuchinger, M., Schwarzfischer, P., Rehberg, T., Reinders, J., Kohler, C. W., Gronwald, W., ... & Spang, R.
Molecular signatures that can be transferred across different omics platforms.
Bioinformatics, 2017; 33(14), i333-i340.
Members of the group
Currently the following people work together
to achieve our aims
- Michael Altenbuchinger, W2-Professor
- Stefan Schrod (PhD)
- Nicole Seifert (PhD)
- Matthias Herb (Research Assistant)
- Jonas Lippl (Research Assistant)
Former members of the group
- Robin Kosch (Postdoc)
- Niklas Lück (Master)
Bachelor- or Master thesis
We offer different topics for Bachelor- or Master thesis in this field.
If you are interessed, please contact:
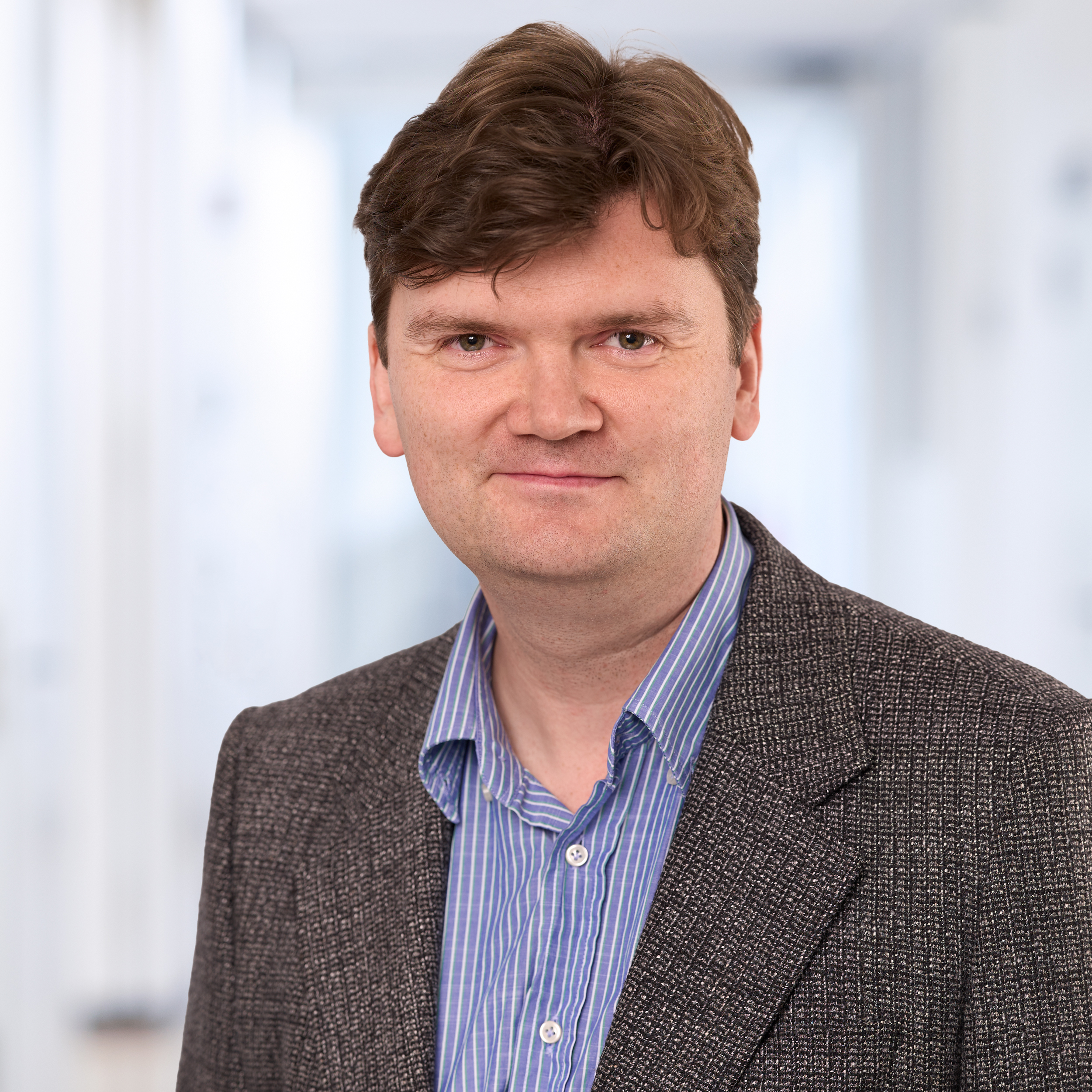
contact information
- telephone: +49 551 3961788
- e-mail address: michael.altenbuchinger(at)bioinf.med.uni-goettingen.de
- location: 2.116
contact information
- telephone: +49 551 3961781
- e-mail address: daniela.grossmann(at)bioinf.med.uni-goettingen.de
- location: 2.107